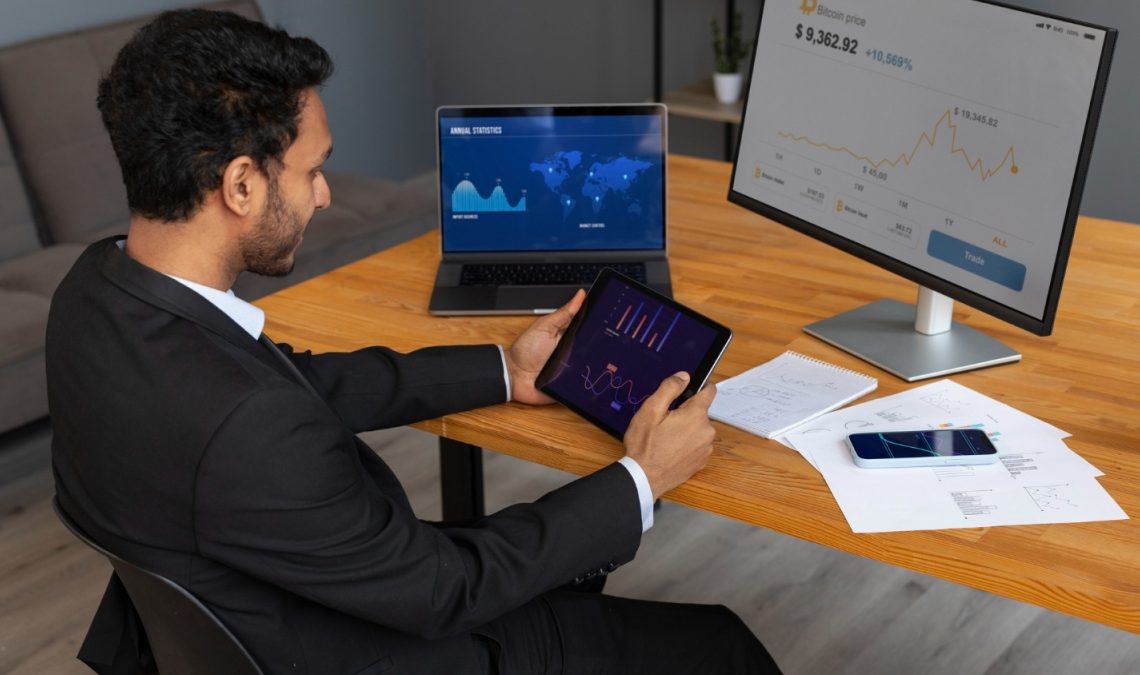
In the fast-evolving world of finance, the rise of technology has transformed traditional trading methods, leading to the emergence of algorithmic trading, a cornerstone of quantitative finance. But what is quantitative finance, and how does it empower algorithmic trading? Let’s explore the intricacies of this field, examine how algorithmic trading works, and understand why it plays a pivotal role in today’s financial markets.
Understanding Quantitative Finance
Quantitative finance is a discipline that uses mathematical models, statistical techniques, and algorithms to analyze financial markets and optimize investment strategies. At its core, quantitative finance applies principles from mathematics, computer science, and finance to understand market dynamics, assess risks, and make data-driven decisions. Professionals in this field, known as “quants,” design and test models that forecast price movements, calculate potential returns, and assess risks associated with various investment strategies. This quantitative approach contrasts with traditional finance, which often relies on qualitative analyses, market sentiment, and intuition.
Answering the question, what is quantitative finance, means delving into a multifaceted field that touches everything from risk management to derivative pricing, and notably, algorithmic trading—a practice transforming the landscape of modern finance.
What is Algorithmic Trading?
Algorithmic trading, often called “algo trading,” involves using pre-programmed algorithms to execute trades automatically. These algorithms are designed to identify trading opportunities, execute trades at optimal times, and manage the volume and frequency of trades, all with minimal human intervention. By leveraging the speed and efficiency of computers, algorithmic trading can capitalize on market inefficiencies and generate profits faster and more accurately than human traders.
At its core, algorithmic trading utilizes various quantitative techniques to analyze historical and real-time data, identify patterns, and develop predictive models. The algorithms can vary in complexity, ranging from simple moving averages to sophisticated machine learning models that adapt based on changing market conditions.
Key Techniques in Algorithmic Trading
Algorithmic trading strategies are grounded in statistical analysis and mathematical models, with some of the most popular techniques being:
- Statistical Arbitrage: This technique identifies pricing inefficiencies between assets by analyzing historical price correlations and exploiting short-lived deviations. Traders can profit from these opportunities by buying undervalued assets and selling overvalued ones, expecting prices to converge.
- Mean Reversion: Based on the principle that asset prices tend to revert to a long-term average, mean reversion models predict price movements by identifying deviations from this average. Algorithms using this approach buy assets when they are underpriced and sell them when they are overpriced, capitalizing on their expected return to the mean.
- Trend Following: Trend-following strategies analyze price trends and momentum to predict future price directions. By following established trends, these algorithms look for upward or downward momentum in asset prices and make trades accordingly, often benefiting from persistent market movements.
- Machine Learning and AI Models: Advanced algorithms increasingly use machine learning to adapt to changing market conditions. These models analyze vast amounts of historical and real-time data to improve accuracy in predicting asset price movements. Unlike traditional models, machine learning algorithms can “learn” from data and adjust their strategies over time.
- High-Frequency Trading (HFT): This approach involves executing a large number of trades within fractions of a second, profiting from small price discrepancies across multiple markets. High-frequency trading requires ultra-low latency, advanced computational power, and access to market data feeds to succeed.
The Advantages of Algorithmic Trading
Algorithmic trading offers numerous advantages over traditional trading approaches. Some of these include:
- Speed and Efficiency: Algorithms can analyze vast datasets, identify opportunities, and execute trades in milliseconds. This speed allows traders to capitalize on fleeting market inefficiencies that would be impossible for human traders to exploit.
- Reduced Human Error: Automated trading removes emotional biases and cognitive errors that can impact decision-making in traditional trading. With algorithms in control, trades are executed based on data and pre-determined rules rather than emotion or impulse.
- Enhanced Accuracy: Algorithmic models can process complex data and deliver precise insights, improving the accuracy of trade execution and minimizing slippage costs.
- Scalability: Algorithms can handle large volumes of data and execute multiple trades simultaneously across different markets and asset classes, making it easier to diversify portfolios and manage risks.
Challenges and Risks of Algorithmic Trading
While algorithmic trading offers significant benefits, it also comes with risks and challenges:
- Market Volatility: Automated trades executed at high speeds can amplify market volatility. Rapid selling triggered by algorithms can lead to “flash crashes,” where prices plummet temporarily due to high sell volumes.
- Model Risk: Algorithms rely on assumptions and historical data to predict market movements, which may not always hold in real-world conditions. If market dynamics change or if there is a flaw in the model, it can lead to significant losses.
- Regulatory Scrutiny: With the growth of algorithmic trading, regulators are increasingly concerned about its impact on market stability. Many jurisdictions have imposed strict rules to prevent market abuse and ensure fair trading practices.
The Future of Algorithmic Trading in Quantitative Finance
As financial markets continue to evolve, algorithmic trading is poised to play an even more prominent role. Technological advancements in machine learning, artificial intelligence, and cloud computing will continue to drive innovation, enabling more sophisticated algorithms that can process larger datasets and adapt to changing conditions in real-time. Additionally, as more trading moves to electronic platforms, the demand for quants and quantitative finance professionals will grow, further establishing what quantitative finance can offer in terms of both opportunity and innovation.
In the future, we may see the emergence of hybrid models that combine algorithmic strategies with human oversight, offering the best of both worlds—automated efficiency with strategic human input. This blend of automation and human intelligence could potentially create a new era of trading where algorithms handle the heavy lifting, and human traders make high-level, strategic decisions.
Conclusion
Quantitative finance has transformed how we think about financial markets, investment strategies, and trading practices, with algorithmic trading at the heart of this transformation. Answering what is quantitative finance in the context of algorithmic trading highlights a data-driven, systematic approach to financial markets that capitalizes on advanced mathematical models and technology. As we look to the future, the ongoing development of algorithmic trading holds promise for even greater efficiency, sophistication, and innovation in finance, continuing to reshape the industry and open new frontiers for investors and traders alike.