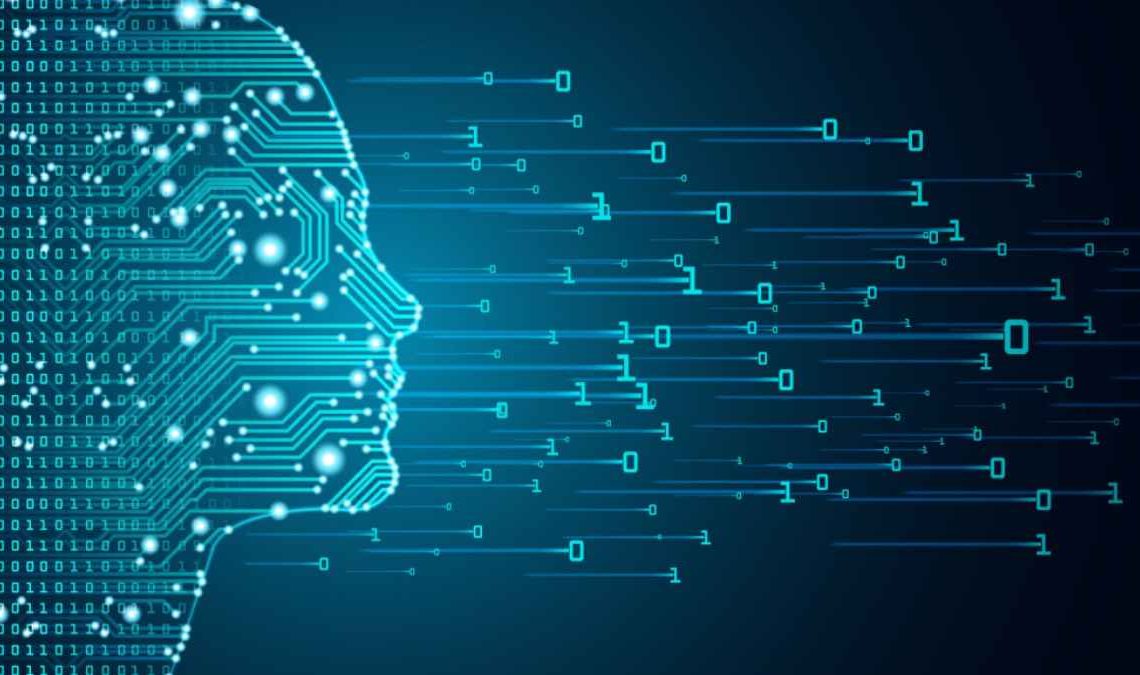
Artificial intelligence analytics is a subfield of AI that employs machine learning methods to mine data for hidden meaning. Artificial intelligence analytics is the act of automating the tasks that a data analyst would otherwise perform. While it’s not the intention, AI analytics can significantly enhance a data analyst’s efficiency, scalability, and visibility into data without replacing them. According to Gartner, augmented analytics is another type of analytics that will become increasingly important in the future. To find patterns in data or uncover valuable insights without data scientists’ help, augmented analytics uses AI and ML.
When Compared to Conventional Analytics, How Do AI Methods Fare?
Let’s look at how artificial intelligence analytics differs from more conventional forms of data analysis. In the past, a specialized group of data analysts often carried out specific data analysis task. To illustrate how a group of analysts may approach a business problem in the past, consider the following scenario:
- Something happens over time at the company; quarterly sales may be lower than expected.
- The next step is for data analysts to speculate on the root causes of the sales slump.
- To determine whether or not the data for that period can support a particular hypothesis, researchers generate ideas and test them against the data.
- After conducting their research, the analysts will draft a report detailing their findings and recommending the subsequent measures for the company to follow.
- Investigating a change and pinpointing its origins takes a lot of time. The solutions found by data analysts are likewise not guaranteed to be correct because of their constraints.
The area of analytics, once driven by centuries-old statistical modeling approaches, is now being revolutionized by artificial intelligence and machine learning, which provide insights at a speed, size, and granularity that are not conceivable for humans. On the other hand, AI analytics built on machine learning algorithms keep a continual eye on and analyze massive data sets. The findings differ from conventional analytics in the ways that:
1. Scale
Without being instructed what to look for, an AI-based anomaly detection solution learns the typical behavior of the data. It can do this at any level of detail, including sales by country, product, sales channel, etc.
2. Speed
The AI model can monitor revenue trends and flag any drops in productivity. A issue’s root cause can be determined via an AI-based analytics solution, allowing for faster problem resolution. This is accomplished through the use of clustering and correlation algorithms. As opposed to quarterly, monthly, or weekly at best with traditional analytics, the time required for remedial action is dramatically decreased due to the continuing nature and real-time nature of the investigation.
3. Accuracy
To give correlations between anomalies that are practically impossible for an analyst to complete (correlations between millions of time series in some situations), AI Analytics is based on ML algorithms that can learn many distinct patterns of normal behavior exceptionally effectively. Since ML algorithms must independently and accurately learn in various ways, it is necessary to employ multiple methods to ensure their accuracy. Our three-part white paper goes into greater depth on these prerequisites.
Unlike human data analysts, these algorithms are unbiased in their approach to the business questions at hand. Artificial intelligence (AI) analytics, for instance, may evaluate enormous amounts of data and provide a completely objective appraisal of the issue, eliminating the need for any preexisting assumptions about the potential causes of a shift in income. This means that artificial intelligence analytics can evaluate endless hypotheses, frequently in seconds rather than weeks.
4. Real-World Applications of AI in Analytics
Now that we’ve covered what AI analytics is and how it differs from traditional data analytics solutions let’s look at some of the ways practically any organization can put AI analytics to work for them.
One of the most potent uses of AI is in demand forecasting, an application of predictive analytics. McKinsey Digital found that using AI for predicting might cut down on mistakes in supply chain networks by as much as 30-50%.
To better forecast when repairs may be necessary, businesses increasingly turn to artificial intelligence (AI)-based solutions known as “predictive maintenance.”
Keeping tabs on Businesses: Almost every business may use diagnostic analytics like this to boost customer happiness, decrease churn, and enhance revenue, as it can be applied to a wide range of business processes (from customer experience monitoring to revenue and cost monitoring).
Modern Analytics Stack Benefits from AI’s Competitive Edge
As shown, using AI for business analytics provides speed, scale, and granularity benefits that are impossible to obtain using traditional data analysts alone. Creating hypotheses, preprocessing data, visualizing information, and using statistical methods are all tasks that traditionally require a lot of human effort in traditional analytics. The problem with this method is that it takes too much time in today’s fast-paced business environment to conduct these duties manually. Many businesses are turning to AI analytics for help with everything from demand forecasting to spotting unusual patterns in their operations. For example, you can employ machine learning algorithms to improve your technical team’s responsiveness to market shifts.